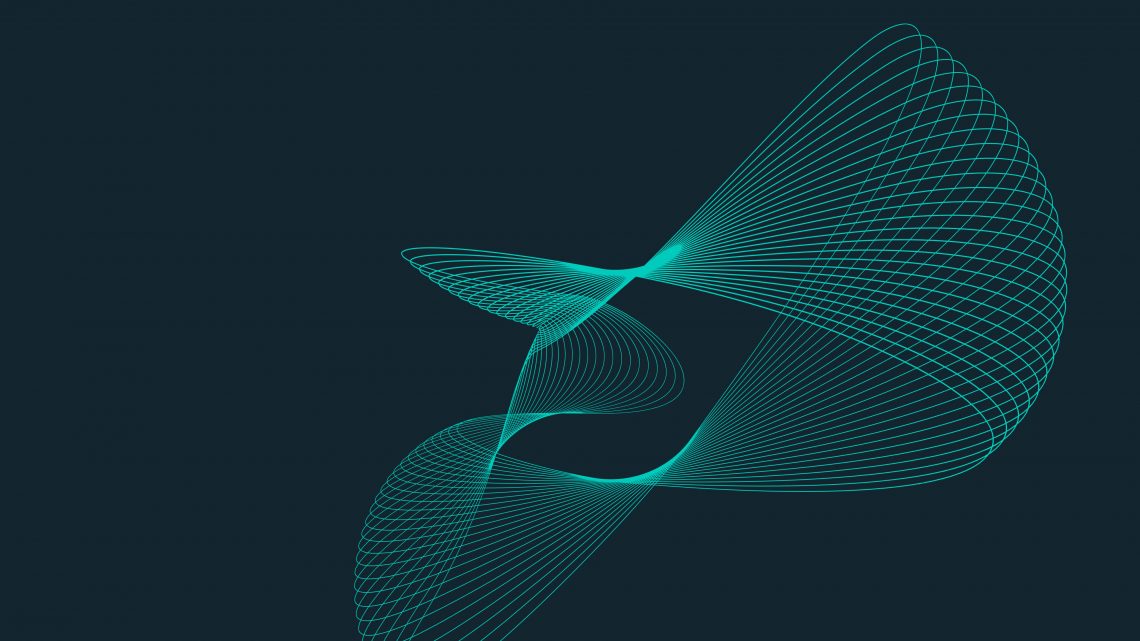
Sustainability through technology
Industrie. Zero
Under the title ‘Industrie.Zero’, the it’s OWL competence network with over 220 members is developing solutions for the digital and sustainable transformation of SMEs.
it's OWL - that's OWL
it’s OWL offers you
Pioneering work for the future of German SMEs
it’s OWL – we are OstWestfalenLippe. Pioneers for Industrie.Zero and Artificial Intelligence. We offer a strong network for SMEs and and link arms with them to tackle the challenges ahead. In short, we are an engine for the competitiveness of industry in OstWestfalenLippe.
Support for topics such as digitalisation, AI and sustainability
We support companies with all questions and topics relating to digitalisation, artificial intelligence and sustainability through technology – from initial information and training courses to concrete implementation projects. Free of charge.
A strong network for the SME sector
Family-owned companies and a strong SME sector form the core of the network. Including numerous global market leaders such as Benteler, Claas, Diebold Nixdorf, DMG Mori, Dr. Oetker, GEA, Hettich and Miele, as well as many hidden champions such as Kannegiesser and WP Kemper. Beckhoff, Harting, KEB, Lenze, Phoenix Contact, Wago and Weidmüller set world standards in industrial electronics. They and over 200 other companies work with universities and research institutes within the cluster. Small to medium-sized companies benefit from new technologies of our core partner companies and research institutions.
Cutting-edge research with application
The universities and research institutions in OstWestfalenLippe stand for interdisciplinary cutting-edge research in areas such as artificial intelligence, automation, future of work and systems engineering. Together with our companies, they jointly develop new technologies and solutions in projects to tackle specific industry challenges.
it's OWL - it's the latest
What's up in OWL
it's OWL perfoms
Our projects
Innovation from OstWestfalenLippe
it’s OWL stands for innovation, research expertise and technology transfer. In our projects, companies and research institutions work together to develop solutions to tackle specific challenges facing industry.
No matching posts found.
Inside it’s OWL
Our digital events
Live webinars with experts from our network and our projects on current topics.
New technologies, current trends and exclusive insights: at ‘Inside it’s OWL’, we take a look at our projects, introduce our topics and connect you with our experts. Digitally on every second Wednesday from 4 to 5 pm.
Your first steps into the network
This is exclusive
Pool of ideas, network and online marketplace: our innovation platform
Exchange ideas, network with experts and benefit from the applications and innovations of our competence network.
Any questions?
What is it's OWL?
Whether it’s the energy transition, a shortage of skilled workers, geopolitical changes or fragile supply chains – industry in Germany is facing major challenges. We offer solutions. it’s OWL: the competence network for Industrie.Zero.
In the Leading-Edge Cluster it’s OWL – Intelligent Technical Systems OstWestfalenLippe – over 220 companies, research institutions and organisations are developing solutions for intelligent products and processes so that companies can produce and work more sustainably. For more than ten years, we have been developing solutions and applications in around 500 projects on topics such as artificial intelligence and intelligent product development, thus supporting companies with digitalisation. These and other competencies lay the foundation for our success as a ‘competence network for Industrie.Zero’. We want to make OstWestfalenLippe a model region for sustainable value creation.
What does 'Industrie.Zero' mean?
Under the title ‘Industrie.Zero’, we are turning OstWestfalenLippe into a model region for sustainable value creation. To this end, we are developing new technologies and applications with the support of the state of North Rhine-Westphalia. For the reduction of CO2 emissions and circular value creation. For intelligent energy supply and sustainable product development. And for new forms of skilled worker development.
How can I benefit from it's OWL as a company?
From initial information and training courses to concrete implementation projects at your company: we offer companies support with all questions and topics relating to digitalisation, artificial intelligence and sustainability through technology.
Which companies are active at it's OWL?
Family-owned companies and a strong SME sector form the core of the network. These include numerous global market leaders: strong brands such as Benteler, Claas, Diebold Nixdorf, DMG Mori, Dr. Oetker, GEA, Hettich and Miele, but also many hidden champions such as Kannegiesser and WP Kemper. Beckhoff, Harting, KEB, Lenze, Phoenix Contact, Wago and Weidmüller set world standards in industrial electronics.
The regional universities and research institutions stand for interdisciplinary cutting-edge research in areas such as artificial intelligence, industrial automation, future of work and systems engineering. Around 1,000 scientists are working on tomorrow’s solutions in four collaborative research centers, 18 research institutes and three Fraunhofer institutes.
How can I get in touch with it's OWL?
The it’s OWL Clustermanagement GmbH team will be happy to answer any questions you may have. Simply get in touch with the contact person for the relevant field.